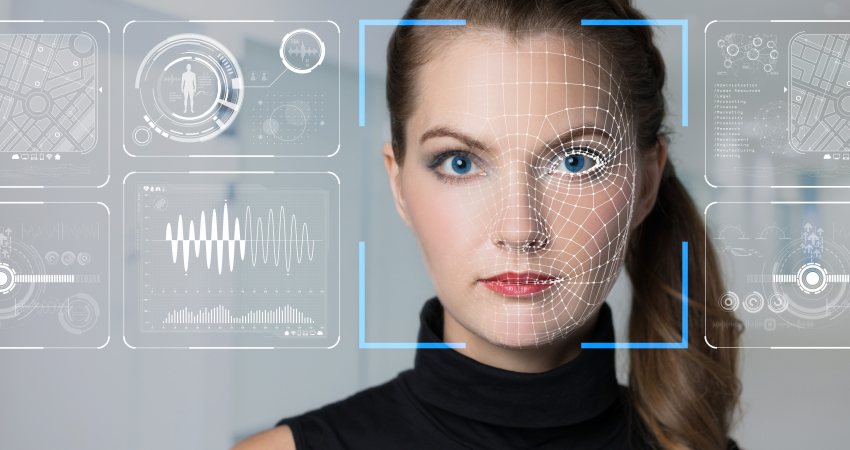
The report identifies five dimensions of the deepfake lifecycle that policy-makers could take into account to prevent and address the, adverse impacts of deepfakes. The legislative framework on artificial intelligence (AI) proposed by the European Commission presents an opportunity to mitigate some of these risks, although regulation should not focus on the technological dimension of deepfakes alone. The report includes policy options under each of the five dimensions, which could be incorporated into the AI legislative framework, the proposed European Union digital services act package and beyond. A combination of measures will likely be necessary to limit the risks of deepfakes, while harnessing their potential.
This report has been written at the request of the Panel for the Future of Science and Technology (STOA) and managed by the Scientific Foresight Unit, within the Directorate-General for Parliamentary Research Services (EPRS) of the Secretariat of the European Parliament.
Downloads
Downloads
-
Report
-
STOA options brief
Summary
This publication is also available via the European Parliament Research Service
Introduction
The emergence of a new generation of digitally manipulated media has given rise to considerable worries about possible misuse. Advancements in artificial intelligence (AI) have enabled the production of highly realistic fake videos, that depict a person saying or doing something they have never said or done. The popular and catch-all term that is often used for these fabrications is 'deepfake', a blend of the words 'deep learning' and 'fake'. The underlying technology is also used to forge audio, images and texts, raising similar concerns.
Recognising the technological and societal context in which deepfakes develop, and responding to the opportunity provided by the regulatory framework around AI that was proposed by the European Commission, this report aims at informing the upcoming policy debate.
The following research questions are addressed:
- What is the current state of the art and five-year development potential of deepfake techniques? (Chapter 3)
- What does the societal context in which these techniques arise look like? (Chapter 4)
- What are the benefits, risks and impacts associated with deepfakes? (Chapter 5)
- What does the current regulatory landscape related to deepfakes look like? (Chapter 6)
- What are the remaining regulatory gaps? (Chapter 7)
- What policy options could address these gaps? (Chapter 8)
The findings are based on a review of scientific and grey literature, and relevant policies, combined with nine expert interviews, and an expert review of the policy options.
Deepfake and synthetic media technologies
In this report, deepfakes are defined as manipulated or synthetic audio or visual media that seem authentic, and which feature people that appear to say or do something they have never said or done, produced using artificial intelligence techniques, including machine learning and deep learning.
Deepfakes can best be understood as a subset of a broader category of AI-generated 'synthetic media', which not only includes video and audio, but also photos and text. This report focuses on a limited number of synthetic media that are powered by AI: deepfake videos, voice cloning and text synthesis. It also includes a brief discussion on 3D animation technologies, since these yield very similar results and are increasingly used in conjunction with AI approaches.
Deepfake video technology
Three recent developments caused a breakthrough in image manipulation capabilities. First, computer vision scientists developed algorithms that can automatically map facial landmarks in images, such as the position of eyebrows and nose, leading to facial recognition techniques. Second, the rise of the internet – especially video- and photo-sharing platforms – made large quantities of audio-visual data available. The third crucial development is the increase in image forensics capacities, enabling automatic detection of forgeries. These developments created the pre-conditions for AI technologies to flourish. The power of AI lies in its learning cycle approach. It detects patterns in large datasets and produces similar products. It is also able to learn from the outputs of forensics algorithms, since these teach the AI algorithms what to improve upon in the next production cycle.
Two specific AI approaches are commonly found in deepfake programmes: Generative Adversarial Networks (GANs) and Autoencoders. GANs are machine learning algorithms that can analyse a set of images and create new images with a comparable level of quality. Autoencoders can extract information about facial features from images and utilise this information to construct images with a different expression (see Annex 3 for further information).
Voice cloning technology
Voice cloning technology enables computers to create an imitation of a human voice. Voice cloning technologies are also known as audio-graphic deepfakes, speech synthesis or voice conversion/swapping. AI voice cloning software methods can generate synthetic speech that is remarkably similar to a targeted human voice. Text-to-Speech (TTS) technology has become a standard feature of everyday consumer electronics, such as Google Home, Apple Siri and Amazon Alexa and navigation systems.
The barriers to creating voice clones are diminishing as a result of a variety of easily accessible AI applications. These systems are capable of imitating the sound of a person's voice, and can 'pronounce' a text input. The quality of voice clones has recently improved rapidly, mainly due to the invention of GANs (see Annex 3).
Thus, the use of AI technology gives a new dimension to voice clone credibility and the speed at which a credible clone can be created. However, it is not just the sound of a voice that makes it convincing. The content of the audio clip also has to match the style and vocabulary of the target. Voice cloning technology is therefore connected to text synthesis technology, which can be used to automatically generate content that resembles the target's style.
Text synthesis technology
Text synthesis technology is used in the context of deepfakes to generate texts that imitate the unique speaking style of a target. The technologies lean heavily on natural language processing (NLP). A scientific discipline at the intersection of computer science and linguistics, NLP's primary application is to improve textual and verbal interactions between humans and computers.
Such NLP systems can analyse large amounts of text, including transcripts of audio clips of a particular target. This results in a system that is capable of interpreting speech to some extent, including the words, as well as a level of understanding of the emotional subtleties and intentions expressed. This can result in a model of a person's speaking style, which can, in turn, be used to synthesise novel speech.
Detection and prevention
There are two distinct approaches to deepfake detection: manual and automatic detection. Manual detection requires a skilled person to inspect the video material and look for inconsistencies or cues that might indicate forgery. A manual approach could be feasible when dealing with low quantities of suspected materials, but is not compatible with the scale at which audio-visual materials are used in modern society.
Automatic detection software can be based on a (combination of) detectable giveaways, some of which are AI-based themselves:
- Speaker recognition
- Voice liveness detection
- Facial recognition
- Facial feature analysis
- Temporal inconsistencies
- Visual artefacts
- Lack of authentic indicators
The multitude of detection methods might look reassuring, but there are several important cautions that need to be kept in mind. One caution is that the performance of detection algorithms is often measured by benchmarking it against a common data set with known deepfake videos. However, studies into detection evasion show that even simple modifications in deepfake production techniques can already drastically reduce the reliability of a detector.
Another problem detectors face is that audio-graphic material is often compressed or reduced in size when shared on online platforms such as social media and chat apps. The reduction in the number of pixels and artefacts that sound and image compression create can interfere with the ability to detect deepfakes.
Several technical strategies may prevent an image or audio clip from being used as an input for creating deepfakes, or limit its potential impact. Prevention strategies include adversarial attacks on deepfake algorithms, strengthening the markers of authenticity of audio-visual materials, and technical aids for people to more easily spot deepfakes.
Societal context
Media manipulation and doctored imagery are by no means new phenomena. In that sense, deepfakes can be seen as just a new technological expression of a much older phenomenon. However, that perspective would fall short when it comes to understanding its potential societal impact. A number of connected societal developments help create a welcoming environment for deepfakes: the changing media landscape by means of online sharing platforms; the growing importance of visual communication; and the growing spread of disinformation. Deepfakes find fertile ground in both traditional and new media because of their often sensational nature. Furthermore, popular visual-first social media platforms such as Instagram, TikTok and SnapChat already include manipulation options such as face filters and video editing tools, further normalising the manipulation of images and videos. Concerningly, non-consensual pornographic deepfakes seem to almost exclusively target women, indicating that the risks of deepfakes have an important gender dimension.
Deepfakes and disinformation
Deepfakes can be considered in the wider context of digital disinformation and changes in journalism. Here, deepfakes are only the tip of the iceberg, shaping current developments in the field of news and media. These comprise phenomena and developments including fake news, the manipulation of social media channels by trolls or social bots, or even public distrust of scientific evidence.
Deepfakes enable different forms of misleading information. First, deepfakes can take the form of convincing misinformation; fiction may become indistinguishable from fact to an ordinary citizen. Second, disinformation – misleading information created or distributed with the intention to cause harm – may be complemented with deepfake materials to increase its misleading potential. Third, deepfakes can be used in combination with political micro-targeting techniques. Such targeted deepfakes can be especially impactful. Micro-targeting is an advertising method that allows producers to send customised deepfakes that strongly resonate with a specific audience.
Perhaps the most worrying societal trend that is fed by the rise of disinformation and deepfakes is the perceived erosion of trust in news and information, confusion of facts and opinions, and even 'truth' itself. A recent empirical study has indeed shown that the mere existence of deepfakes feeds distrust in any kind of information, whether true or false.
Benefits, risks and impacts
Deepfake technologies can be used for a wide variety of purposes, with both positive and negative impacts. Beneficial applications of deepfakes can be conceived in the following areas: audio-graphic productions; human-machine interactions (improving digital experiences); video conferencing; satire; personal or artistic creative expression; and medical (research) applications (e.g. face reconstruction or voice creation).
Deepfake technologies may also have a malicious, deceitful and even destructive potential at an individual, organisational and societal level. The broad range of possible risks can be differentiated into three categories of harm: psychological, financial and societal. Since deepfakes target individual persons, there are firstly direct psychological consequences for the target. Secondly, it is also clear that deepfakes can be created and distributed with the intent to cause a wide range of financial harms. Thirdly, there are grave concerns about the overarching societal consequences of the technology.
An overview of the risks identified in this research are presented in the table on page IV of the report.
Cascading impacts
The impact of a single deepfake is not limited to a single type or category of risk, but rather to a combination of cascading impacts at different levels (see infographic below). First, as deepfakes target individuals, the impact often starts at the individual level. Second, this may cause harm to a specific group or organisation. Third, the notion of the existence of deepfakes, a well-targeted deepfake, or the cumulative effect of deepfakes, may lead to severe harms on the societal level.
The infographic depicts three scenarios that illustrate the potential impacts of three types of deepfakes on the individual, group and societal levels: a manipulated pornographic video; a manipulated sound clip given as evidence; and a false statement to influence the political process.
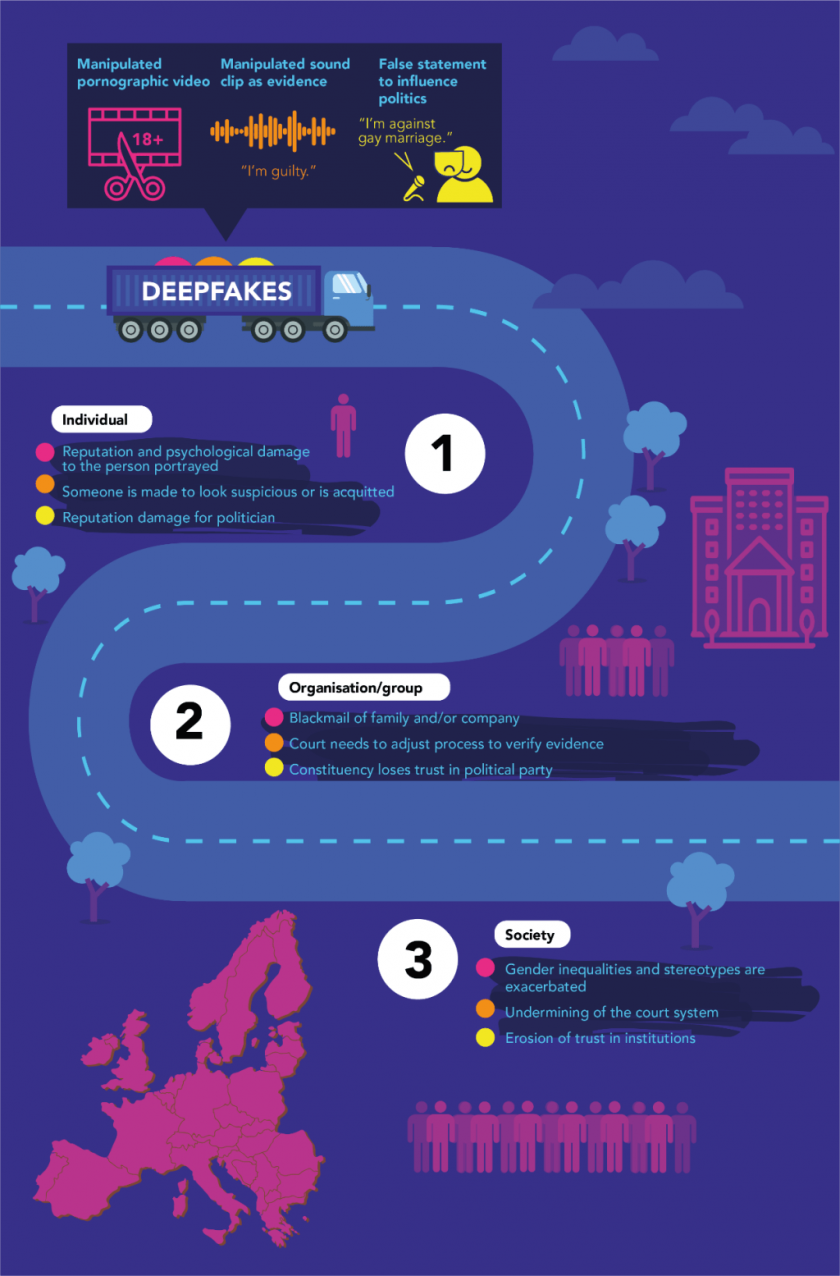
Conclusion
This research has identified numerous malicious as well as beneficial applications of deepfake technologies. These applications do not strike an equal balance, as malicious applications pose serious risks to fundamental rights. Deepfake technologies can thus be considered dual-use and should be regulated as such.
The invention of deepfake technologies has severe consequences for the trustworthiness of all audio-graphic material. It gives rise to a wide range of potential societal and financial harms, including manipulation of democratic processes, and the financial, justice and scientific systems. Deepfakes enable all kinds of fraud, in particular those involving identity theft. Individuals – especially women – are at increased risk of defamation, intimidation and extortion, as deepfake technologies are currently predominantly used to swap the faces of victims with those of actresses in pornographic videos.
Taking an AI-based approach to mitigating the risks posed by deepfakes will not suffice for three reasons. First, other technologies can be used to create audio-graphic materials that are effectively similar to deepfakes. Most notably 3D animation techniques may create very realistic video footage.
Second, the potential harms of the technology are only partly the result of the deepfake videos or underlying technologies. Several mechanisms are at play that are equally essential. For example, for the manipulation of public opinion, deepfakes need not only to be produced, but also distributed. Frequently, the policies of media broadcasters and internet platform companies are instrumental to the impact of deepfakes.
Third, although deepfakes can be defined in a sociological sense, it may prove much more difficult to grasp the deepfake videos, as well as the underlying technologies, in legal terms. There is an inherent subjective aspect to the seeming authenticity of deepfakes. A video that may seem convincing to one audience, may not be so to another, as people often use contextual information or background knowledge to make a judgement about authenticity.
Similarly, it may be practically impossible to anticipate or assess whether a particular technology may or may not be used to create deepfakes. One has to bear in mind that the risks of deepfakes do not solely lie in the underlying technology, but largely depend on its use and application. Thus, in order to mitigate the risks posed by deepfakes, policy-makers could consider options that address the wider societal context, and go beyond regulation. In addition to the technological provider dimension, this research has identified four additional dimensions for policy-makers to consider: deepfake creation; circulation; target/victim; and audience.
The overall conclusion of this research is that the increased likelihood of deepfakes forces society to adopt a higher level of distrust towards all audio-graphic information. Audio-graphic evidence will need to be confronted with higher scepticism and have to meet higher standards. Individuals and institutions will need to develop new skills and procedures to construct a trustworthy image of reality, given that they will inevitably be confronted with deceptive information. Furthermore, deepfake technology is a fast-moving target. There are no quick fixes. Mitigating the risks of deepfakes thus requires continuous reflection and permanent learning on all governance levels. The European Union could play a leading role in this process.
Policy options
This section is also available as pdf via the download button at the upper right corner of the page
Tackling deepfakes in European policy
Novel artificial intelligence (AI) and other contemporary digital advances have given rise to a new generation of manipulated media known as deepfakes. Their emergence is associated with a wide range of psychological, financial and societal impacts occurring at individual, group and societal levels. The Panel for the Future of Science and Technology (STOA) requested a study to examine the technical, societal and regulatory context of deepfakes and to develop and assess a range of policy options, focusing in particular upon the proposed AI (AIA) and digital services acts (DSA), as well as the General Data Protection Regulation (GDPR). This briefing summarises the policy options developed in the study. They are organised into five dimensions – technology, creation, circulation, target and audience – and are complemented by some overarching institutional measures.
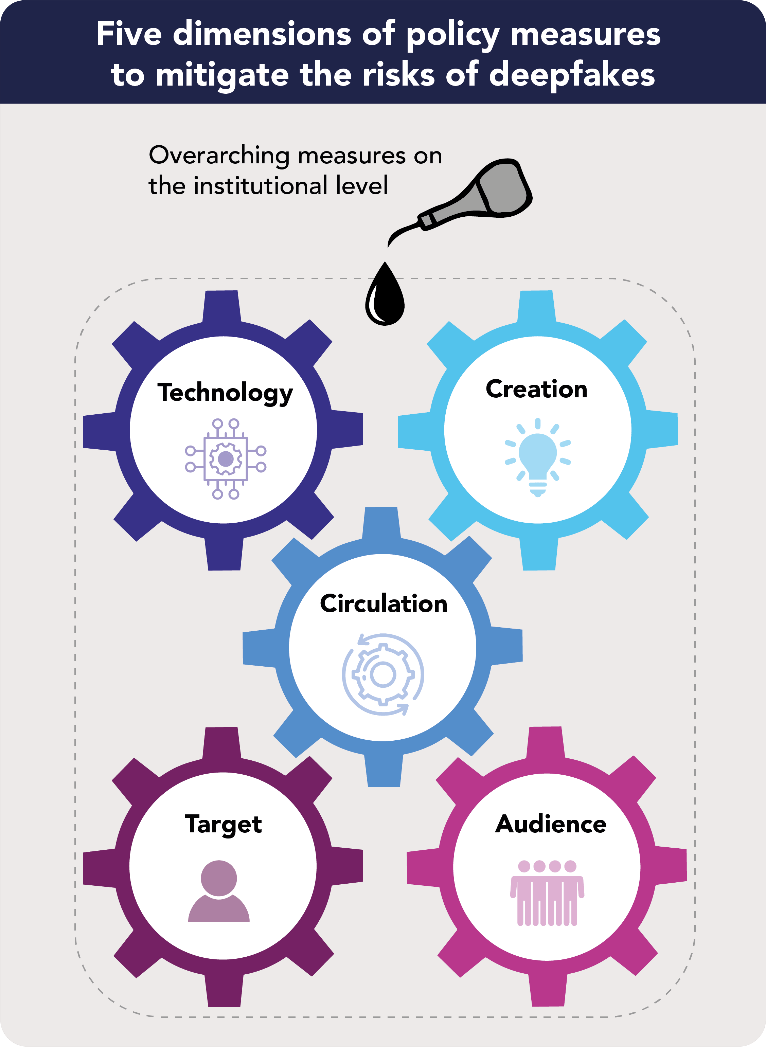
Technology dimension
The technology dimension concerns the underlying technologies and tools that are used to generate deepfakes, and the actors that develop deepfake production systems.
Clarify which AI practices should be prohibited under the AIA: The proposed AIA mentions four types of prohibited AI practices that could relate to certain applications of deepfake technology. However, the formulation of these sections is open to interpretation. Some deepfake applications appear to fulfil the criteria of high-risk applications, such as enabling deceptive manipulation of reality, inciting violence or causing violent social unrest.
Create legal obligations for deepfake technology providers: As proposed, the AIA would not oblige technology providers to label deepfake content, so the responsibility for labelling deepfakes currently lies with deepfake creators. The AIA could be extended to oblige the producers of deepfake creation tools to incorporate labelling features.
Regulate deepfake technology as high risk: Deepfake applications of AI could be defined as high risk by including them in annex III of the proposed AIA. This may be justified by risks to fundamental rights and safety, a criterion that is used to determine whether AI systems are high risk. Doing so would place explicit legal requirements on the providers of deepfake technologies, including risk-assessment, documentation, human oversight and ensuring high-quality datasets.
Limit the spread of deepfake detection technology: While detection technology is crucial in halting the circulation of malicious deepfakes, knowledge of how they work can help deepfake producers to circumvent detection. Limiting the diffusion of the latest detection tools could give those that possess them an advantage in the 'cat-and-mouse game' between deepfake production and detection. However, limiting detection technology to too narrow a group of actors could also restrict others from legitimate use.
Invest in the development of AI systems that restrict deepfake attacks: While technology solutions cannot address all deepfake risks, mechanisms such as Horizon Europe could be mobilised to invest in the development of AI systems that prevent, slow, or complicate deepfake attacks.
Invest in education and raise awareness amongst IT professionals: Familiarity with the impacts of deepfakes (and other AI applications) could become a standard part of the curriculum for information technology professionals, in particular AI researchers and developers. This may also provide an opportunity to equip them with a greater understanding and appreciation of the ethical and societal impacts of their work, as well as the legal standards and obligations in place.
Creation dimension
While the technology dimension concerns the production of deepfake generation systems, the creation dimension concerns those that actually use such systems to produce deepfakes. Those that do so for malicious purposes may actively evade identification and enforcement efforts.
Clarify the guidelines for labelling: While standardised labels may help audiences to identify deepfakes, the proposed AIA does not state what information should be provided in the labels, or how it should be presented.
Limit the exceptions for the deepfake labelling requirement: The proposed AIA places a labelling obligation on users of deepfake technology. However, it also creates exemptions when deepfakes are used for law enforcement, in arts, sciences, and where the use 'is needed for freedom of expression'. Liberal interpretation of these exceptions may allow many deepfakes to remain un-labelled.
Ban certain applications: Transparency obligations alone may be insufficient to address the severe negative impacts of specific applications of deepfakes such as non-consensual deepfake pornography or political disinformation campaigns. While an outright ban may be disproportionate, certain applications could be prohibited, as seen in some jurisdictions including the United States of America, the Netherlands and the United Kingdom. Given the possible strong manipulative effect of deepfakes in the context of political advertising and communications, a complete moratorium on such applications could be considered. However, any such bans should be sensitive to potential impacts upon freedom of expression.
Diplomatic actions and international agreements: The use of disinformation and deepfakes by foreign states, intelligence agencies and other actors contributes to increasing geopolitical tension. While some regional agreements are in place, there are no binding global agreements to deal with information conflicts and the spreading of disinformation. Intensified diplomatic actions and international cooperation could help to prevent and de-escalate such conflicts, and economic sanctions could be considered when malicious deepfakes are traced back to specific state actors.
Lift some degree of anonymity for using online platforms: Anonymity serves as protection for activists and whistle-blowers, but can also provide cover for malicious users. Users of online platforms in China need to register with their identity (ID). If some degree of platform anonymity is considered essential, more nuanced approaches could require users to identify themselves before uploading certain types of content, but not when using platforms in other ways.
Invest in knowledge and technology transfer to developing countries: The negative impacts of deepfakes may be stronger in developing countries. Embedding deepfake knowledge and technology transfer into foreign and development policies could help improve these countries' resilience.
Circulation dimension
Policy options in the circulation dimension are particularly relevant in the context of the proposed DSA, which provides opportunities to limit the dissemination and circulation of deepfakes and, in doing so, to reduce the scale and the severity of their impact.
Detecting deepfakes and authenticity: Platforms and other intermediaries could be obliged to embed deepfake detection software and enforce labelling, or to detect the authenticity of users to counteract amplification in the dissemination of deepfakes and disinformation.
Establish labelling and take-down procedures: Platforms could be obliged to label content detected as a deepfake and to remove it when notified by a victim or trusted flagger. This could be done transparently, under human oversight, and with proper notification and appeal procedures. A distinction could be made between reporting by any person and reporting by persons directly affected.
Limit platforms' decision-making authority decide unilaterally on the legality and harmfulness of content: Independent oversight of content moderation decisions could limit the influence of platforms on freedom of expression and the quality of social communication and dialogue.
Increase transparency: To support monitoring activities, the DSA's reporting obligations could be extended to include deepfake detection systems, their results and any subsequent decisions.
Slow the speed of circulation: While freedom of speech is a fundamental right, freedom of reach is not. Platforms could be obliged to slow the circulation of deepfakes by limiting the number of users in groups, the speed and dynamics of sharing patterns, and the possibilities for micro-targeting.
Target dimension
Malicious deepfakes can have severe impacts on targeted individuals, and these may be more profound and long-lasting than many traditional patterns of crime.
Institutionalise support for victims of deepfakes: National advisory bodies could provide accessible judicial support to help victims ensure take-downs, identify perpetrators, launch civil or criminal proceedings, and access psychological support. They could also contribute to the long-term monitoring of deepfakes and their impacts.
Strengthen the capacity of data protection authorities (DPAs) to respond to the use of personal data for deepfakes: Since deepfakes tend to make use of personal data, DPAs could be equipped with specific resources to respond to the challenges they raise.
Provide guidelines on GDPR in the context of deepfakes: DPAs could develop guidelines on how the GDPR framework applies to deepfakes, including the circumstances in which a data protection impact assessment is required and how freedom of expression should be interpreted in this context.
Extend the list of special categories of personal data with voice and facial data: The GDPR could be extended to include voice and facial data, to specify the circumstances under which their use is permitted and clarify how freedom of expression should be interpreted in the context of deepfakes.
Develop a unified approach for the proper use of personality rights. Personality rights are comprised of many different laws including various rights of publicity, privacy and dignity. The 'right to the protection of one's image' could be developed and clarified in light of deepfake developments.
Protect personal data of deceased persons. Deepfakes can present deceased persons in misleading ways without their consent. A 'data codicil' could be introduced to help people control how their data and image is used after their death.
Address authentication and verification procedures for court evidence: Various types of digital evidence, such as electronic seals, time stamps and electronic signatures, have been established as admissible as evidence in legal proceedings. Guidelines could be provided to help address authentication and verification issues and support courts when dealing with digital evidence of questionable authenticity.
Audience dimension
Audience response is a key factor in the extent to which deepfakes can transcend the individual level and have wider group or societal impacts.
Establish authentication systems: In parallel to labelling measures, authentication systems could help recipients of messages to verify their authenticity. These could require raw video data, digital watermarks or information to support traceability.
Invest in media literacy and technological citizenship: Awareness and literacy of deepfake technologies could increase the resilience of citizens, organisations and institutions against the risks of deepfakes. These could target different profiles, such as young children, professionals, journalists and social media users.
Invest in a pluralistic media landscape and high quality journalism: The European democracy action plan recognised a pluralistic media landscape as a prerequisite for access to truthful information, and to counter disinformation. Support for journalism and media pluralism at European and national levels could help maintain this.
Institutional and organisational measures
Overarching options for institutional and organisational action could support and complement measures in all five dimensions discussed above.
Systematise and institutionalise the collection of information with regards to deepfakes: Systemic collection and analysis of data about the development, detection, circulation and impact of deepfakes could inform the further development of policies and standards, enable institutional control of deepfake creation, and may even transform deepfake creation culture. This option corresponds with the European democracy action plan, the European action plan against disinformation and the European Digital Media Observatory that is currently being formed. The European Union Agency for Cybersecurity (ENISA) and European Data Protection Board (EDPB) could also play a role in this.
Protecting organisations against deepfake fraud: Organisations could be supported to perform risk assessments for reputational or financial harm caused by malicious deepfakes, to prepare staff and establish appropriate strategies and procedures.
Identify weaknesses and share best practices: Assessments of national regulations in the context of deepfakes could reveal weaknesses to be addressed, as well as best-practices to be shared. An EU-wide comparative study could be promoted within the framework of Horizon Europe.