The precautionary principle and clinical decision support systems in healthcare
Photo: Shutterstock
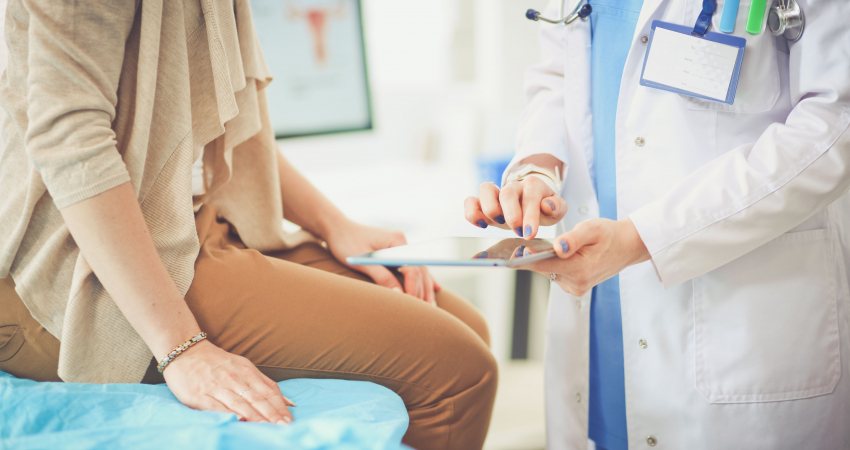
When there is uncertainty about the risks of innovative products, precaution must be applied. What does applying precaution mean for the prospects of an innovation? What does applying the precautionary principle mean in practice? In this article, we demonstrate the relevance of the precautionary principle in the use of clinical decision support systems in healthcare.
In short:
- Clinical decision support systems in healthcare could have great benefits, but they are also accompanied by considerable, uncertain risks.
- We show that in some cases, a precautionary approach to the use of this technology in healthcare is desirable.
- The precautionary principle allows for important considerations. For example, when it comes to the limits that should be set on the use of these systems.
Imagine: you have just had a serious accident. Your condition is critical. The medical staff rushes you to the operating theatre. The assistant tells you that the doctor is on the way. The doctor will have to make a decision about the necessary treatment. This decision will be crucial for the long-term damage of the accident. After a few minutes of waiting, out of the corner of your eye, you see a white coat fluttering around the corner. Thank goodness. The doctor has arrived, opens a laptop and, aloud, asks it what to do. And a robotic voice says what needs to be done.
Of course, the scenario above is not (yet) a reality. However, make no mistake, machines programmed with medical knowledge are already playing an increasingly important role in healthcare decision-making. Clinical decision support systems, using artificial intelligence, are already 'thinking' along with medical staff. For example, they warn healthcare workers in the case of unusual readings, provide guidance during treatment or make suggestions about actions to be taken.
These clinical decision support systems (CDSS) are supposed to facilitate the work of medical professionals or even improve their actions. The use of these systems makes decision-making faster and more accurate, and reduces the number of human errors, or so the promise goes. Greater efficiency and effectiveness of medical decisions should also lead to lower healthcare costs. The technical preconditions for these advantages are that the data used by these systems must be complete and correct. In many cases, there are doubts as to whether decisions can be made for individual cases on the basis of large data sets, for example for individuals with complex or multiple disorders. In addition, the algorithms used must meet safety standards. For example, not everyone should be able to modify the algorithm just like that.
Besides these technical considerations, there are also concerns and uncertainties about the practical use of these decision support systems. How far can these systems go in their support? Do they not, in some cases, replace the decision-making of care workers too much? And what are the consequences for the patient?
As we have shown in previous articles on precaution and innovation, the precautionary principle is often used in the event of serious but uncertain risks. In this paper, we describe why a precautionary approach is also important in clinical decision support systems, and what such an approach might look like in practice.
RECIPES
This article is based on one of the ten case studies in the RECIPES project. The purpose of these case studies is to gain more insight into the controversies and complexities involved in applying the precautionary principle to various innovations
The results of the RECIPES project will allow the EU to remain at the forefront of science by re-examining the precautionary principle in relation to innovation and major societal challenges. The project started in January 2019 and will last for three years, with an extension of six months. Eleven organisations from seven European countries are working together on the RECIPES project. The initiator of this consortium is the Faculty of Law at Maastricht University.
The risks of clinical decision support systems
Between different clinical decision support systems, there is considerable variation in the risks they could pose for the healthcare sector. The negative consequences of a defective system used to advise on policy in the event of an outbreak, are of course many times greater than those of a system that merely supports a GP assistant in referring a potential patient. Nevertheless, in a general sense, a number of similar risks can be identified. We will discuss these below.
There are inherent risks in making treatment decisions within the medical sector. This is also the case when using clinical decision support systems. Within a hospital, for example, countless decisions are made every day that determine the health or life expectancy of people. Think of choices about physical interventions and medication needed after a certain diagnosis. The consequences of such decisions are often not 100 % certain, and there is always a certain risk involved.
In addition, clinical decision support systems also involve new risks, because their use changes the way decisions are made. And it is precisely this that can pose additional risks. For example: decision-making becomes dehumanised, there is a lack of clarity in the division of responsibilities, and certain biases in decisions go unnoticed.
Risks and consequences
Firstly, there is the risk that clinical decision support systems can dehumanise decision-making. After all, a machine is not a human being. The decisions of these systems are based on data and computational logic. This differs from the (implicit) knowledge and experience of care workers. It is impossible to translate years of experience, intuition, socio-emotional intelligence and the sense of context into machine language. Especially in the case of psychosocial problems, it can be important for a GP to inquire about a patient's personal circumstances. Depression, for example, can have deeper causes - such as loneliness, debts, relational problems - that require a human perspective and dialogue.
Delegating decision making to machines (or being supported by machines) also implies a loss of control for caregivers. As a result, the division of responsibilities may become unclear. If a medical decision is largely determined by an algorithm, the control of the doctor in question decreases. To what extent is the treatment advice still the doctor's decision? Can the doctor shift part of the responsibility to the algorithm or its developer? When the advice of these systems is leading, the developers and maintenance staff will bear responsibility for this. After all, they will have insight into how the system makes a medical decision based on the input data. And they will know how to update the system on the basis of new, medical knowledge.
In addition, there is a risk of discrimination. When an algorithm is developed on the basis of big data, existing discrimination in care systems, or in the data collection in the decision systems, can be built into it without anyone noticing. There are already examples where intelligent systems made decisions that were severely disadvantageous to women and ethnic minorities. Many medical datasets are based mainly on men's data, so some AI systems make decisions that do not fit women's bodies that well. And in the United States, a biased algorithm reduced access to health care for African-American people.¹
Scientific uncertainty
So far, little research has been done into the risks of clinical decision support systems. There are roughly three reasons for this:
First of all, these kinds of AI-based systems are complex and their processes are unpredictable, especially in the case of unsupervised machine learning² Researchers warn, for example, of cyber attacks that can alter the behaviour of unsupervised machine learning AI systems by slightly tweaking the data.³
A second reason for the uncertainty about risks lies in the environment in which decision support systems are applied. The environment in which healthcare takes place, such as a hospital, is characterised by a high degree of complexity, unpredictability and ambiguity. In order to work properly and safely, the systems must be attuned to all the specific (changing) protocols, norms, standards and practices of different employees. Also, the systems must function in the context of a physician's daily work, the specific needs of a patient, and the supervision of a manager and/or a privacy officer.
A third reason is related to the high demands of a good decision. It is difficult to estimate whether such systems can meet these high demands. A good decision must be transparent, explainable and supported by relevant data. A good decision must also be based on sufficient reflection and take into account the privacy, autonomy and dignity of the patient. These are subjective requirements that are open to different interpretations. This makes it difficult to assess when such systems are 'sufficiently' capable of making good decisions.
Applying the precautionary principle to the use of artificial intelligence in healthcare
How should the precautionary principle be applied when using clinical decision support systems in healthcare?
This question is difficult to answer, as there is a great deal of variation within these systems. Nevertheless, the precautionary principle ('better safe than sorry') does seem relevant in some cases. A (temporary) ban on the use of such systems is a logical consequence. After all, careless development and use of these systems entail serious, uncertain risks. The systems can have harmful consequences for many people, especially when they are applied on a large scale.
The risks and uncertainties described in this article give an indication of the application of precaution in these systems. The technical causes of uncertain risks show that it is important, for example, to make certain principles - such as transparency - an integral part of the design. The analysis of the environment in which the systems are used shows that, in healthcare, it is particularly important to involve those who will be using the systems at an early stage. Finally, it becomes clear that it is important to have sufficient discussion about the criteria on which support systems should be evaluated. What are the conditions for a good decision in the specific environment where a system will be applied? By answering such questions, and taking measures prior to implementation in a healthcare setting, the precautionary principle can be applied to these systems.
Conclusion: ensure precaution in healthcare
The precautionary principle allows for important considerations. It is important to reflect on serious risks and uncertainties. These reflections can, in turn, provide openings for new innovations that better deal with these concerns. After all, when it comes to the accessibility and stability of healthcare, we do not want to take any unnecessary risks.
The next article in this series will focus on the application of the precautionary principle to gene drives. An overview of the entire series on the precautionary principle can be found below.
¹ The algorithm was used to predict the future health of individuals based on their past medical records. It identified people who were likely to need additional care in the future. For various socio-economic reasons related to access to healthcare, black patients use healthcare less. And therefore generate lower costs than white patients. The algorithm then prioritised white people over black people with the same health status.
Obermeyer, Z. (2019), Dissecting racial bias in an algorithm used to manage the health of populations, Science 25, Vol. 366, Issue 6464, 447-453. DOI: 10.1126/science.aax2342
² Unsupervised machine learning allows the system to discover connections in unstructured and unlabelled data 'by itself'. As a result, it may be impossible for humans to know afterwards what these connections are based on.
³ Changing a few pixels on a lung scan, for example, could fool such a system into detecting a non-existent disease. Finlayson, S. G. et al (2019) Adversarial attacks on medical machine learning, Vol. 363, Issue 6433, 1287-1289.