Innovating with AI in healthcare: 'Get the data in order first'
Illustration: Max Kisman
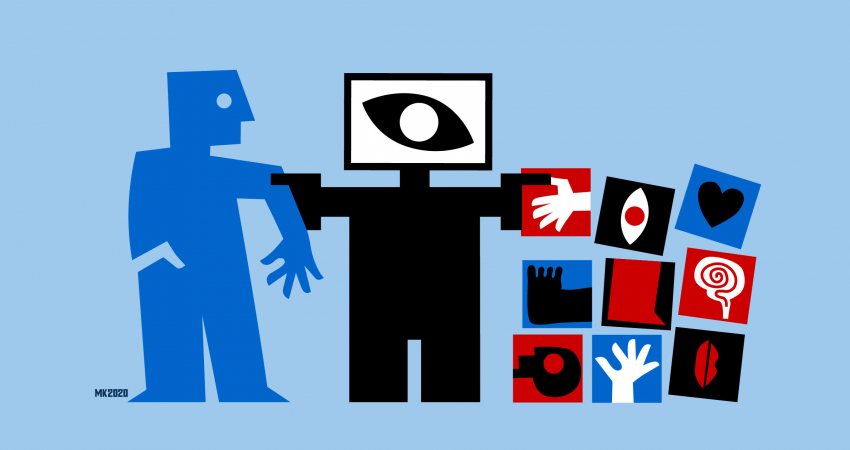
In the blog series 'Healthy Bytes' we investigate how artificial intelligence (AI) can be used responsibly for our health. In this eleventh part, Max Welling, Professor of Machine Learning at the University of Amsterdam and Vice President of Technology at Qualcomm, has the floor. In the future, AI may support doctors in making diagnoses and drawing up a treatment plan. Some algorithms will even be able to outperform doctors at certain tasks. But first, in a European context, we need to get the collection and exchange of data in order, says Max Welling.
In short:
- How is AI used responsibly for our health? That's what this blog series is about.
- According to Max Welling, in order to make real progress with AI in healthcare, we first need to be able to share data in a safe, systematic way.
- There is no reason to think that some form of AI cannot ultimately become as smart as a human being. Things will become possible that we cannot even imagine right now.
Looking for good examples of the application of artificial intelligence in healthcare, we asked key players in the field of health and welfare in the Netherlands about their experiences. In the coming weeks we will share these insights via our website. How do we make healthy choices now and in the future? How do we make sure we can make our own choices when possible? And do we understand in what way others take responsibility for our health where they need to? Even with the use of technology, our health should be central.
An elephant cycling on the moon
AI already sometimes outperforms people. For example, if you ask a clearly formulated and delineated question and you have a large dataset to train an AI system with. However, you only have to go a little outside of the training domain and an AI algorithm gets confused. For example, an algorithm that diagnoses skin cancer. If it is trained on pictures of white skins and you want to apply the algorithm to a dark skin, the algorithm gets stuck. A doctor has a deeper knowledge of skin cancer, so he or she can apply it to both white and dark skin.
This is where AI differs from the intelligence of humans. What a person learns in one context, he or she can apply in another. This is because a person has a lot of background knowledge about the world we live in. Knowledge about natural laws, social laws, culture, cause and effect. We embed new knowledge in our background knowledge and then we can apply it in another context. This makes us much more flexible than AI.
Just think of an elephant cycling on the moon. You probably have an image in your head right now, even though you have never seen that elephant before and you don't think it is possible that you will ever see an elephant cycling on the moon. We humans can combine the three abstract concepts 'elephant', 'bicycle' and 'moon' into one image. An algorithm has difficulty with that. As researchers in machine learning, we are now busy developing systems that are as flexible as humans. And I don't think there's any reason to think that AI can't eventually become as smart as a human being.
AI in the hospital: a lot of data and clear problems
AI systems that can be used in (hospital) care do not even have to be as flexible. Most problems are well defined. For example, only a limited number of illnesses are possible and a doctor in training must learn them all. So it is mainly a matter of correctly classifying the complaints X, Y and Z of the incoming patient. AI, in addition, is able to 'know' many more diseases than a doctor. For example, AI is not tied to a specialisation such as cardiology or urology. Moreover, AI also knows very rare diseases, which a doctor may only encounter once in his or her career.
Innovation in healthcare is now mainly limited by the availability of data. Privacy legislation is in the way. This makes it difficult for us to exchange data. For example, even during the corona crisis, it is not possible to share lung X-rays. However, little privacy-sensitive data can be extracted from such a photograph. It is often technically possible to build privacy into the system, for example by encrypting data and not storing it centrally. The lack of transparency of self-learning AI systems can also be solved technically. It is often not clear to users how a system achieves a certain outcome, such as a diagnosis. An algorithm can provide insight into how the system arrives at a particular diagnosis and thus enable the physician to verify this.
Algorithms can be used in all kinds of applications, good and bad.
Innovate responsibly
As a professor, I am involved in the development of fundamental algorithms. I focus on mathematics. At that stage, I do not consider it relevant to make an ethical assessment. The algorithms can then be used in all kinds of applications, good and bad. Only when you start applying the method in practice do ethical and social considerations become relevant. At that stage, I am certainly prepared to help thinking about robust applications and questions of safety. An algorithm that is very good at predicting, but is not transparent, may be suitable for predicting developments on the financial market. There, it is particularly important that it works well. However, for medical applications it may not be as suitable, because we want to be able to monitor decisions and therefore demand more transparency.
Nevertheless, I do not want to sit in other people's chairs. Lawyers, sociologists and philosophers also have an important role in assessing algorithms in specific application practices. It is my passion to develop the foundations of algorithms behind self-learning systems. It is the task of, for example, an ethics committee and other experts to assess the safety and social impact of possible applications. What I can argue from my perspective is that AI legislation should be flexible. Because technology is developing so rapidly, it is important that laws are not laid down for ten years. I also see a lot in a system of certification of methods. In order to get a certificate, a method must be tested through and through. A logistics system must therefore be built in order to be able to certify safely and quickly. So that you can trust an algorithm even if you do not fully understand what is happening under the bonnet.
AI in healthcare - getting the data in order first
In order to make real progress with AI in health care, we first need to get the data in order. In the medical world a lot of data is collected, but these are not currently available to train algorithms on. This data must therefore be made available in a safe and standardised manner at a European level. It is up to the EU to organise this. That is a major challenge. Once we have that in place, researchers will be able to develop all kinds of algorithms. Perhaps then things will become possible that we cannot even come up with at the moment.
Artificial intelligence in healthcare: deciding together is crucial
Health
03 February 2021
Article
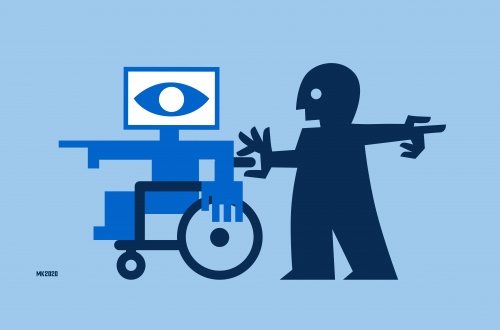
Towards proper management of data technology in healthcare
Health
11 January 2021
Article
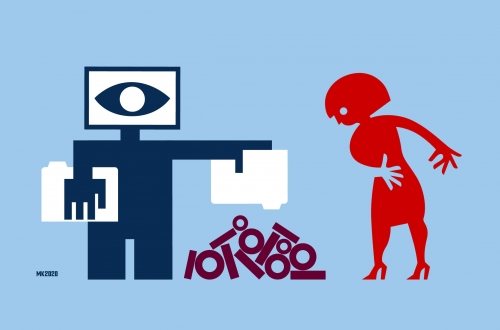
Towards healthy data use for medical research
Health
04 January 2021
Article
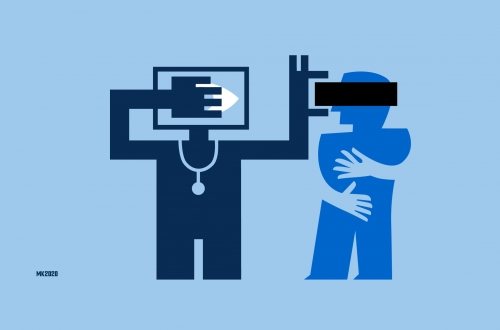
Innovating with AI in healthcare: 'So people can participate in society'
Health
21 December 2020
Article
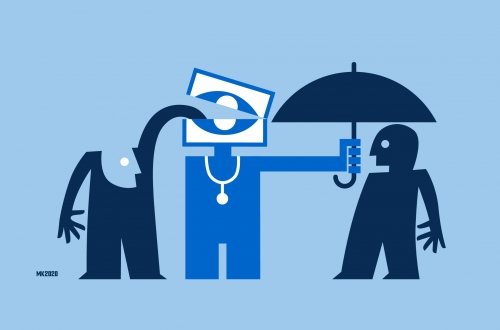
How an investment fund can contribute to responsible AI in healthcare
Health
14 December 2020
Article
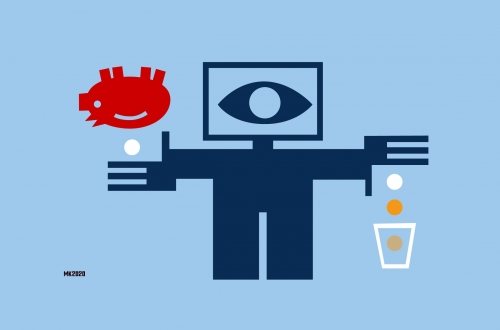
Entrepeneurship with AI in healthcare: need for cooperation and strategy
Health
07 December 2020
Article
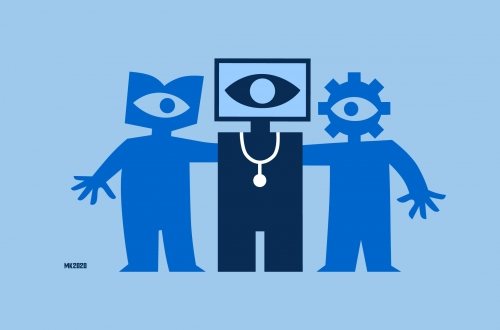
AI in care: implications for education
Health
30 November 2020
Article
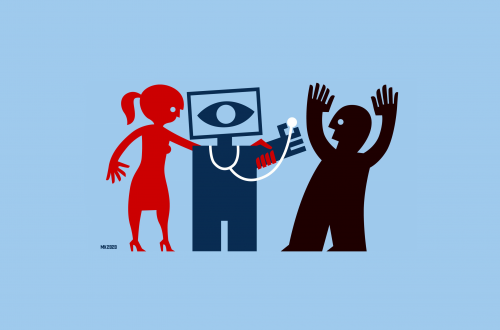
Responsible AI in healthcare: the value of examples
Health
23 November 2020
Article
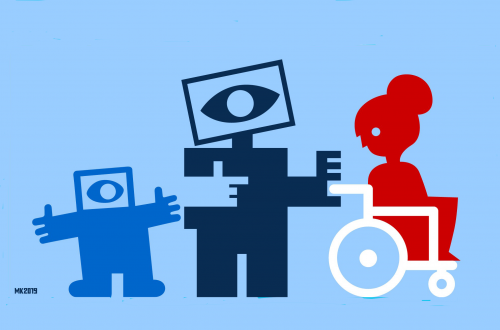
How AI helps a person with dementia eat their sandwich on time
Health
16 November 2020
Article
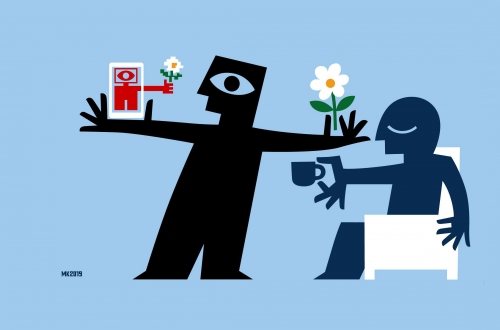
Policy for AI in health care: a balancing of values
Health
09 November 2020
Article
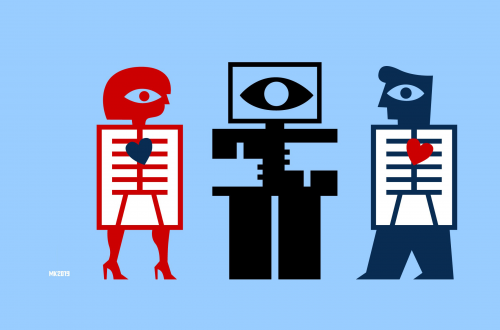
Artificial intelligence in healthcare: who decides?
Health
04 November 2020
Article
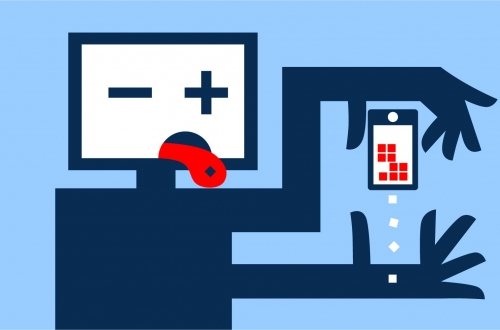