Towards proper management of data technology in healthcare
Illustration: Max Kisman
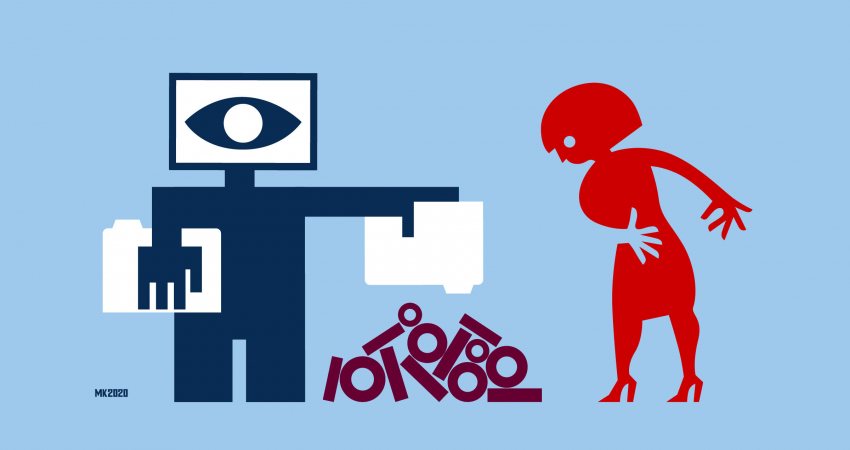
In the blog series 'Healthy Bytes' we investigate how artificial intelligence (AI) can be used responsibly for our health. In this tenth part, Antoinette de Bont, Professor of Sociology of Innovation in Healthcare at Erasmus University Rotterdam, shows that social scientists can contribute to good management of data technology in healthcare. “Why don't we start with a moral data deliberation?”
In short
- How is AI used responsibly for our health? That's what this blog series is about.
- Antoinette de Bont argues that good management of data technology is needed.
- She shows how insights from social scientists can contribute to this.
Looking for good examples of the application of artificial intelligence in healthcare, we asked key players in the field of health and welfare in the Netherlands about their experiences. In the coming weeks we will share these insights via our website. How do we make healthy choices now and in the future? How do we make sure we can make our own choices when possible? And do we understand in what way others take responsibility for our health where they need to? Even with the use of technology, our health should be central.
Successful and legitimate use of AI
Artificial intelligence (AI) can detect patterns in large volumes of data. This enables AI to make predictions better and faster than humans. Take an AI application that analyses a photo of a tumour. Ideally, a doctor with such a photo would no longer need to take a biopsy of the tumour. In this way, the use of AI can lead to less invasive cancer diagnostics.
The risk, however, is that AI will only lead to additional care. Developers of AI applications need to understand why a surgeon might want to cut anyway, even if they have a picture of the tumour. A surgeon may want to feel and smell, for example. If AI is only used alongside existing practice, instead of being included in it, the added value of the technology is small.
So the question is what is needed to utilise AI successfully and legitimately, so that the technology really supports healthcare.
Social scientists
It is necessary for social scientists to be represented among technicians and doctors. They could, for example, clarify what a concept such as 'legitimate use of AI’ means. Legitimacy of the use of technology concerns, for example, whether the use is in accordance with the rules (compliance). But it can also be about social legitimacy: do those who work with the technology know what they are using and why? We also talk about moral legitimacy: are we using AI for the right cause?
There is a lot of focus on compliance, but less on the social and moral legitimacy of AI in healthcare. Without social scientists, questions of legitimacy would not be sufficiently addressed in the development and deployment of AI in healthcare.
In addition, social scientists can contribute to a better understanding of rules and cultural differences in the interpretation of the rules. Anyone who wants to develop and deploy AI in healthcare has to deal with, among other things, the General Data Protection Regulation (GDPR) from the EU, with national rules, and with the rules of the healthcare system in a particular country. Start-ups must find their way through this complex system.
In our research project BigMedilytics (Big Data for Medical Analytics) we have created infographics that make these complex rules more transparent. In this way, it also becomes clear that the interpretation of the GDPR can vary greatly from one country to another. This is an important insight, because those who work with AI are quick to think across borders. The more data, the better. But to really be able to use an AI application, you have to take the local culture and interpretation into account. What is the meaning of privacy in a country?
Many actors and factors
It takes a long time before an AI application can be used. The algorithm must be built into an instrument and the innovation must be tested. Many different parties have to put up with each other for a long time and there has to be money to keep them involved. A business case is needed.
In our BigMedilytics project, we want to draw up models of the relationships between all these actors and factors. Imagine that doctors do not have confidence in a new technology. What consequences does that have for other actors? With such a model, we can analyse what exactly happens and what is needed to put the technology into practice.
There are many questions for further research. For example: what does 'commercial' mean?
Questions for further research
Research and debate are needed on what socially responsible use of data entails. If a commercial company develops an AI application, should patients who make their data available for this purpose receive a discount on the use of that AI application? Have they already paid with their data? Is such a pricing mechanism socially responsible?
Another question: what is 'commercial'? Many patients do not want their data to be used for commercial purposes. That is why a definition of 'commercial' is needed. An AI application to determine the premium for life insurance might clearly fall under 'commercial'. But the development of new medicines or new prevention tools: is that also commercial?
Another question: why do some people allow the use of their data, and others do not? Spain, like Sweden, has the concept of 'data donation'; data donation as a social duty. You should not benefit from the technology without contributing to it yourself. There is an opposite culture in Germany. There the government can, and wants to, intervene to protect citizens against the sharing of data for commercial use.
If the Dutch government uses the word 'data solidarity', then the question is what that means. We need to talk about a balance between individual autonomy and collective responsibility. Empirical research is also valuable in this respect. Is it actually true that people leave a collective system if they have the option of opting out?
Towards proper management of data technology
Data is in the hands of (large) companies; the technology is a fact. Good management, rules and governance structures are now needed to successfully and legitimately deploy AI in healthcare. We need to make agreements on how data can be used.
It is important that people are able to make mistakes. They must learn to deal with the complex rules. Informal mechanisms can be useful. If something has gone wrong, talk to each other so that the underlying cause of the error emerges. Many organisations have already implemented a form of moral deliberation; why not start with a moral data deliberation?
Artificial intelligence in healthcare: deciding together is crucial
Health
03 February 2021
Article
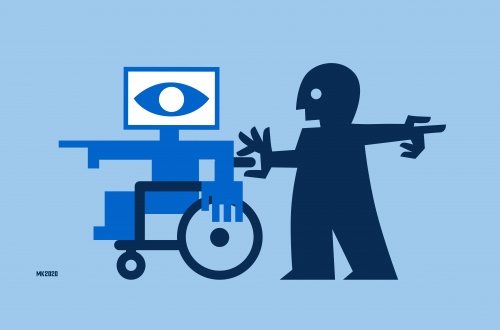
Innovating with AI in healthcare: 'Get the data in order first'
Health
18 January 2021
Article
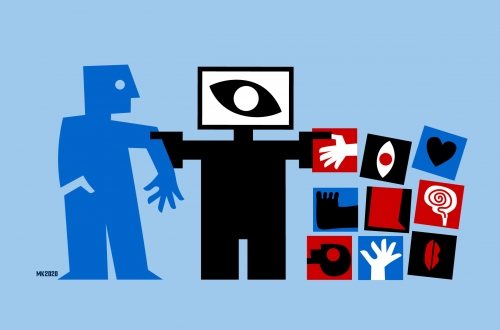
Towards healthy data use for medical research
Health
04 January 2021
Article
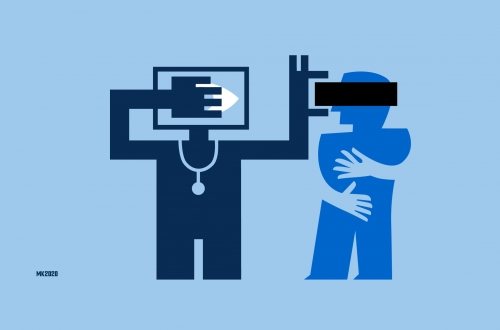
Innovating with AI in healthcare: 'So people can participate in society'
Health
21 December 2020
Article
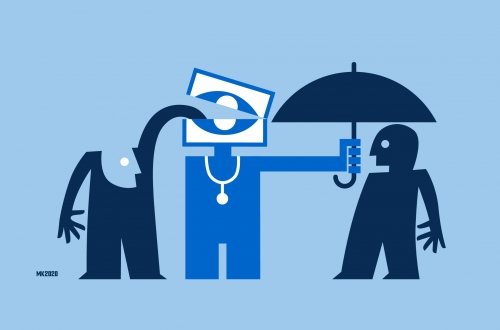
How an investment fund can contribute to responsible AI in healthcare
Health
14 December 2020
Article
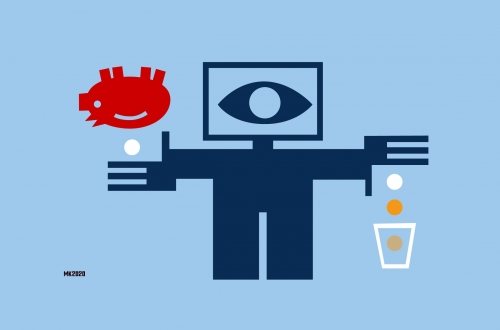
Entrepeneurship with AI in healthcare: need for cooperation and strategy
Health
07 December 2020
Article
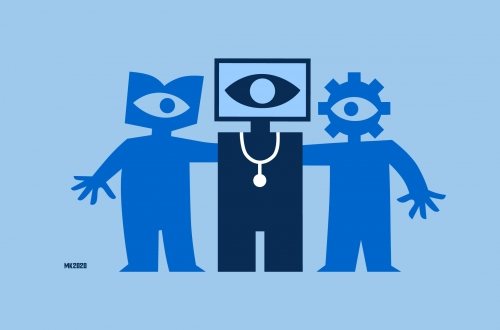
AI in care: implications for education
Health
30 November 2020
Article
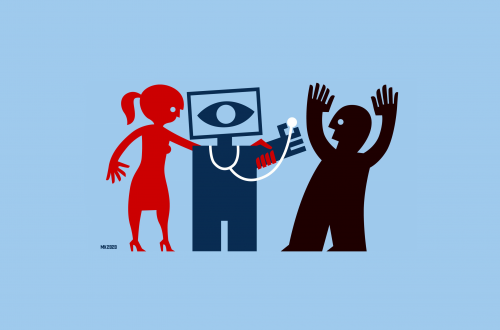
Responsible AI in healthcare: the value of examples
Health
23 November 2020
Article
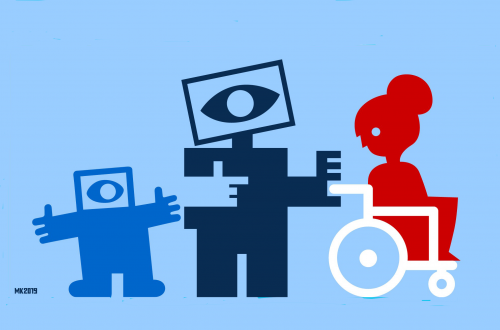
How AI helps a person with dementia eat their sandwich on time
Health
16 November 2020
Article
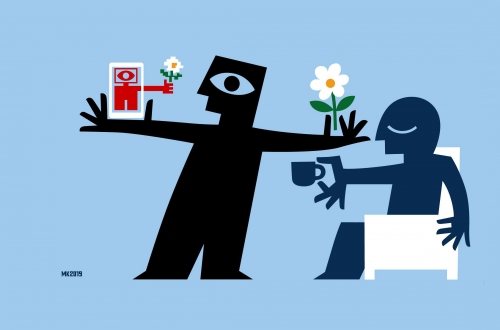
Policy for AI in health care: a balancing of values
Health
09 November 2020
Article
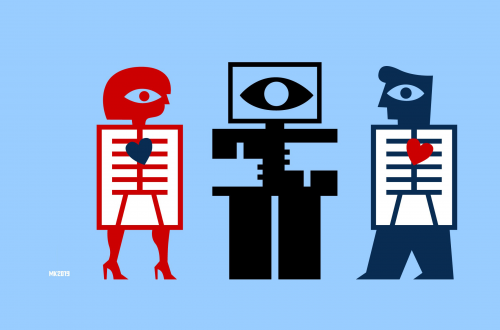
Artificial intelligence in healthcare: who decides?
Health
04 November 2020
Article
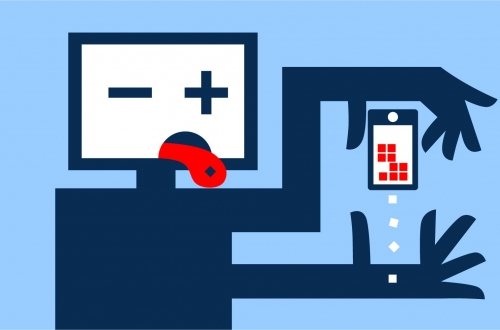